The goal here was to get the synthetic colors in the SDSS, 2MASS and WISE filters of ~2000 model objects generated by the PHOENIX stellar and planetary atmosphere software.
Since it would be silly (and incredibly slow… and much more boring) to just calculate and store every single color for all 12 filter profiles, I wrote a module to calculate colors a la carte.
The Filters
I got the J, H, and K band relative spectral response (RSR) curves in the 2MASS documentation, the u, g, r, i and z bands from the SDSS documentation, and the W1, W2, W3, and W4 bands from the WISE documentation.
I dumped all my .txt filter files into one directory and wrote a function to grab them all, pull out the wavelength and transmission values, and output the filter name in position [0], x-values in [1], and y-values in [2]:
def get_filters(filter_directory):
import glob, os
files = glob.glob(filter_directory+'*.txt')
if len(files) == 0:
print 'No filters in', filter_directory
else:
filter_names = [os.path.splitext(os.path.basename(i))[0] for i in files]
RSR = [open(i) for i in files]
filt_data = [filter(None,[map(float,i.split()) for i in j if not i.startswith('#')]) for j in RSR]
for i in RSR: i.close()
RSR_x = [[x[0] for x in i] for i in filt_data]
RSR_y = [[y[1] for y in i] for i in filt_data]
filters = {}
for i,j,k in zip(filter_names,RSR_x,RSR_y):
filters[i] = j, k, center(i)
return filters
Calculating Apparent Magnitudes
We can’t have colors without magnitudes so here’s a function to grab the Teff and log g specified spectra, and calculate the apparent magnitudes in a particular band:
def mags(band, teff='', logg='', bin=1):
from scipy.io.idl import readsav
from collections import Counter
from scipy import trapz, log10, interp
s = readsav(path+'modelspeclowresdustywise.save')
Fr, Wr = [i for i in s.modelspec['fsyn']], [i for i in s['wsyn']]
Tr, Gr = [int(i) for i in s.modelspec['teff']], [round(i,1) for i in s.modelspec['logg']]
# The band to compute
RSR_x, RSR_y, lambda_eff = get_filters(path)[band]
# Option to specify an effective temperature value
if teff:
t = [i for i, x in enumerate(s.modelspec['teff']) if x == teff]
if len(t) == 0:
print "No such effective temperature! Please choose from 1400K to 4500K in 50K increments or leave blank to select all."
else:
t = range(len(s.modelspec['teff']))
# Option to specify a surfave gravity value
if logg:
g = [i for i, x in enumerate(s.modelspec['logg']) if x == logg]
if len(g) == 0:
print "No such surface gravity! Please choose from 3.0 to 6.0 in 0.1 increments or leave blank to select all."
else:
g = range(len(s.modelspec['logg']))
# Pulls out objects that fit criteria above
obj = list((Counter(t) & Counter(g)).elements())
F = [Fr[i][::bin] for i in obj]
T = [Tr[i] for i in obj]
G = [Gr[i] for i in obj]
W = Wr[::bin]
# Interpolate to find new filter y-values
I = interp(W,RSR_x,RSR_y,left=0,right=0)
# Convolve the interpolated flux with each filter (FxR = RxF)
FxR = [convolution(i,I) for i in F]
# Integral of RSR curve over all lambda
R0 = trapz(I,x=W)
# Integrate to find the spectral flux density per unit wavelength [ergs][s-1][cm-2] then divide by R0 to get [erg][s-1][cm-2][cm-1]
F_lambda = [trapz(y,x=W)/R0 for y in FxR]
# Calculate apparent magnitude of each spectrum in each filter band
Mags = [round(-2.5*log10(m/F_lambda_0(band)),3) for m in F_lambda]
result = sorted(zip(Mags, T, G, F, I, FxR), key=itemgetter(1,2))
result.insert(0,W)
return result
Calculating Colors
Now we can calculate the colors. Next, I wrote a function to accept any two bands with options to specify a surface gravity and/or effective temperature as well as a bin size to cut down on computation. Here’s the code:
def colors(first, second, teff='', logg='', bin=1):
(Mags_a, T, G) = [[i[j] for i in get_mags(first, teff=teff, logg=logg, bin=bin)[1:]] for j in range(3)]
Mags_b = [i[0] for i in get_mags(second, teff=teff, logg=logg, bin=bin)[1:]]
colors = [round(a-b,3) for a,b in zip(Mags_a,Mags_b)]
print_mags(first, colors, T, G, second=second)
return [colors, T, G]
The PHOENIX code gives the flux as Fλ in cgs units [erg][s-1][cm-2][cm-1] but as long as both spectra are in the same units the colors will be the same.
Makin’ It Handsome
Then I wrote a short function to print out the magnitudes or colors in the Terminal:
def print_mags(first, Mags, T, G, second=''):
LAYOUT = "{!s:10} {!s:10} {!s:25}"
if second:
print LAYOUT.format("Teff", "log g", first+'-'+second)
else:
print LAYOUT.format("Teff", "log g", first)
for i,j,k in sorted(zip(T, G, Mags)):
print LAYOUT.format(i, j, k)
The Output
Then if I just want the J-K color for objects with log g = 4.0 over the entire range of effective temperatures, I launch ipython and just do:
In [1]: import syn_phot as s
In [2]: s.colors('J','K', logg=4)
Teff -------- log g -------- J-K
1400.0 ------ 4.0 ---------- 4.386
1450.0 ------ 4.0 ---------- 4.154
...
4450.0 ------ 4.0 ---------- 0.756
4500.0 ------ 4.0 ---------- 0.733
Similarly, I can specify just the target effective temperature and get the whole range of surface gravities. Or I can specify an effective temperature AND a specific gravity to get the color of just that one object with:
In [3]: s.colors('i','W2', teff=3050, logg=5)
Teff -------- log g -------- J-K
3050.0 ------ 5.0 ---------- 3.442
I can also reduce the number of data points in each flux array if my sample is very large. I just have to specify the number of data points to skip with the “bin” optional parameter. For example:
In [4]: s.colors('W1','W2', teff=1850, bin=3)
This will calculate the W1-W2 color for all the objects with Teff = 1850K and all gravities, but only take every third flux value.
I also wrote functions to generate color-color, color-parameter and color-magnitude plots but those will be in a different post.
Plots!
Here are a few color-parameter animated plots I made using my code. Here’s how I made them. Click to animate!
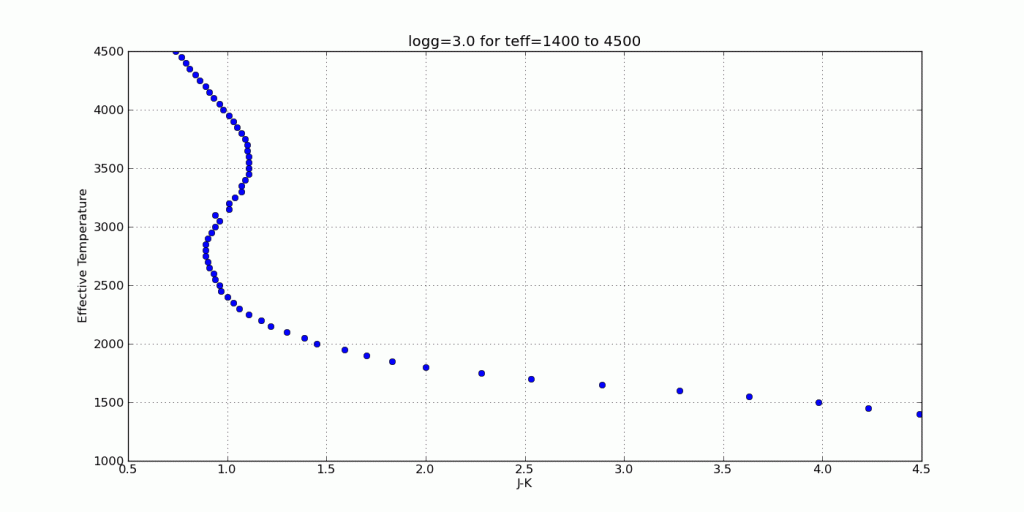
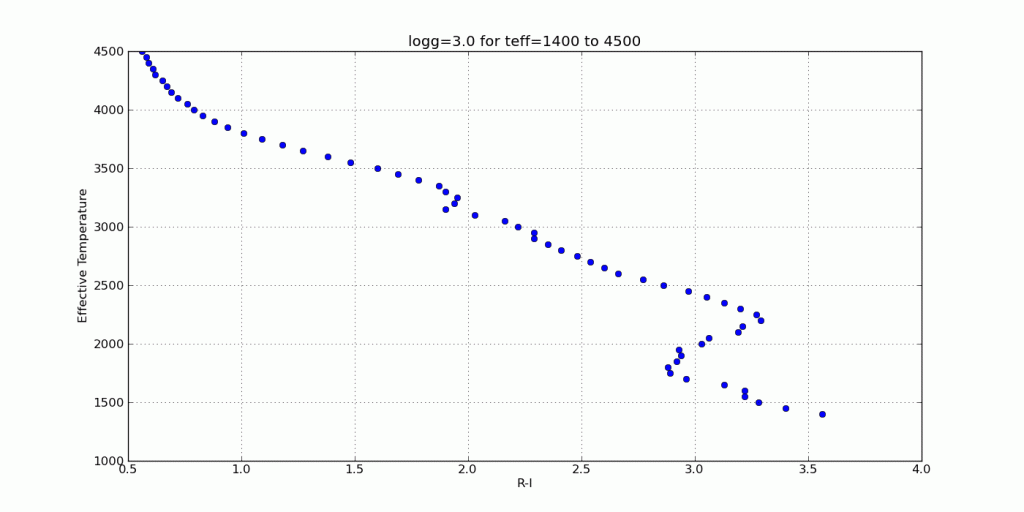
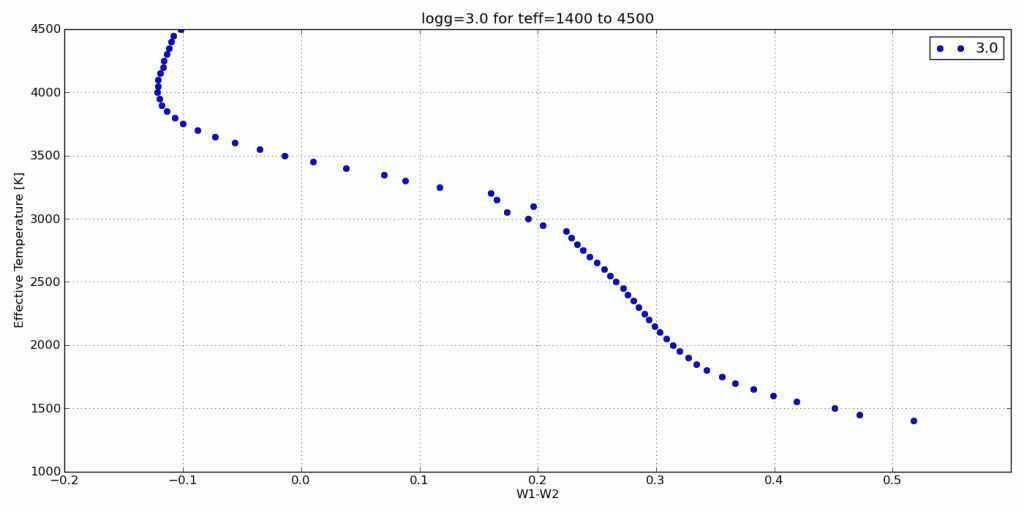
And here are a few colorful-colorful color-color plots I made:
Plots with observational data
Just to be sure I’m on base, here’s a color-color plot of J-H vs. H-Ks for objects with a log surface gravity of 5 dex (blue dots) plotted over some data for the Chamaeleon I Molecular Cloud (semi-transparent) from Carpenter et al. (2002).
The color scale is for main sequence stars and the black dots are probable members of the group. Cooler dwarfs move up and to the right.
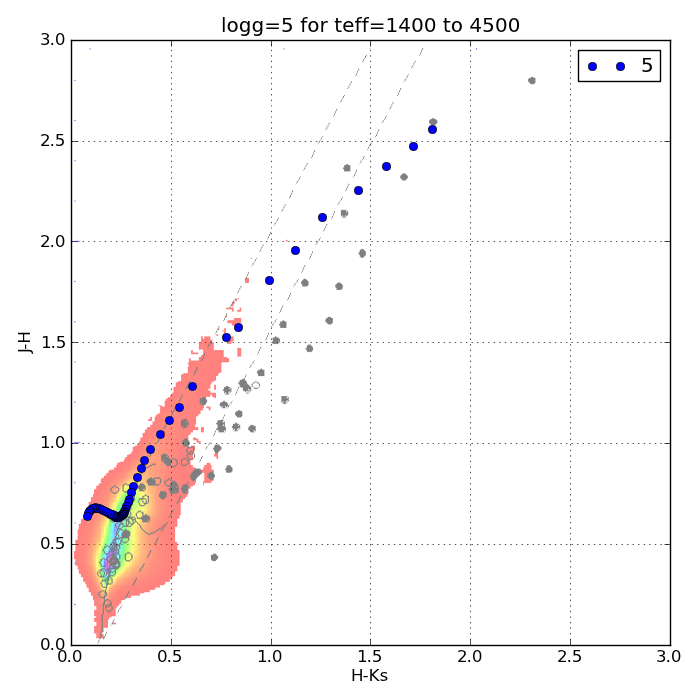
And here’s a plot of J-Ks vs. z-Ks as well as J-Ks vs. z-J. Again, the blue dots are from my synthetic photometry code at log(g)=5 and the semi-transparent points with errors are from Dahn et al. (2002).
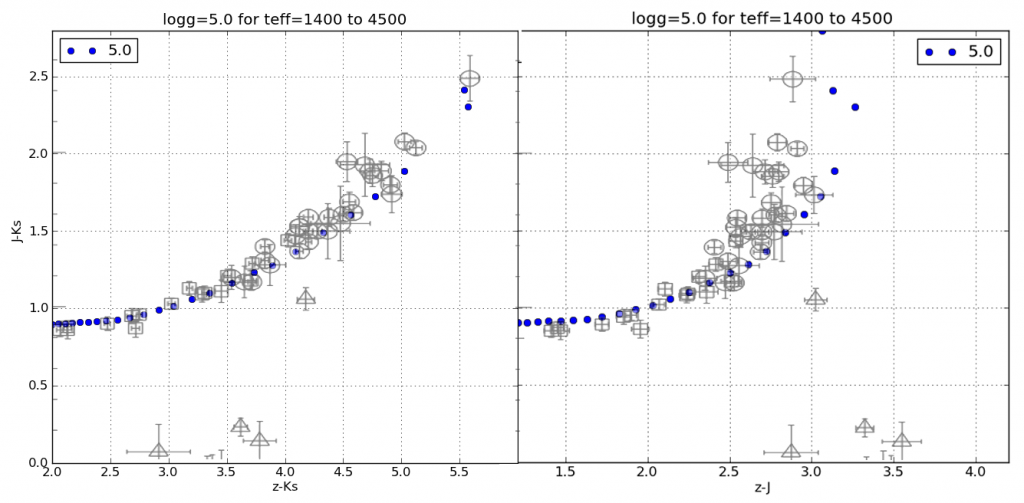